Bridge management system
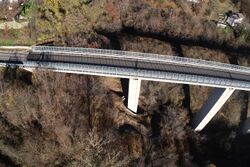
A bridge management system (BMS) is a set of methodologies and procedures for managing information about bridges. Such system is capable of document and process data along the entire life cycle of the structure steps: project design, construction, monitoring, maintenance and end of operation.[1][2]
First used in literature in 1987, the acronym BMS is commonly used in structural engineering to refer to a single or a combination of digital tools and software that support the documentation of every practice related to the single structure.[3] Such software architecture has to meet the needs of road asset managers interested on tracking the serviceability status of bridges through a workflow mainly based on 4 components: data inventory, cost and construction management, structural analysis and assessment and maintenance planning.[1] The implementation of BMS usually is built on top of relational databases, geographic information systems (GIS) and building information modeling platform (BIM) also named bridge information modeling (BrIM)[4] with photogrammetric and laser scanning processing software used for the management of data collected during targeted inspections. The output of the whole procedure, as stated also in some national guidelines of different countries, usually consists of a prioritization of intervention on bridges classified in different risk level according to information collected and processed.[5][4]
History
Since the late 1980s the structural health assessment and monitoring of bridges represented a critical topic in the field of civil infrastructure management.[6] In the 1990s, the Federal Highway Administration (FHWA) of the United States promoted and sponsored PONTIS and BRIDGEIT, two computerized platforms for viaduct inventory and monitoring named BMSs.[2][6] In the following years, also outside the U.S., the growing need of an organized and digitized road asset management has led responsible national agencies to adopt increasingly complex solutions able to meet their objectives, such as building inventories and inspection databases, planning for maintenance, repair and rehabilitation interventions in a systematic way, optimizing the allocation of financial resources, and increasing the safety of bridge users.[1] Moreover, as of 2020s, the occurrence of some significant bridge collapse events and an increased sensitivity to the environmental impact of large structure management operations has led some national authorities such as France and Italy to the designation of national guidelines with detailed guidance for the development and adoption of multilevel BMS to optimize bridge management.[7][8][5]
System components
Researchers in the field of structural engineering have identified 4 main components for the implementation of a functional BMS:[1]
- Data inventory.
- Cost and construction management.
- Structural analysis and assessment.
- Maintenance planning.
Data inventory
While the collection of historical documentation and project design of the structure is represented by analogical and digital archives managed by road asset managers, the geometric data input implies the application of topographic techniques through dedicated surveys on the field. In particular, bridge inspections for the 3D reconstruction of a digital twin of the structure usually consists of survey campaigns using global navigation satellite system measurements, ground and drone-based photogrammetry and laser scanning. Data management in this phase implies the use of geographic information systems, BIM and computer-aided design software, manipulating both 2D and 3D geo-referenced data. Resulting products include point clouds and meshes that serves as the basis for building information modeling processes. Bridge surveys can be repeated in different steps of the structure life cycle and their frequency depends on decision making and prioritization of maintenance operation and national guidelines.[1]
In addition to visual geomatic inspections, other nondestructive evaluation techniques are commonly adopted, allowing to collect data not limited to the accurate geometric reconstruction of the structure but also to the material conditions. In this case, the adoption of ground penetrating radar for detection of deterioration of the reinforcement in decks and infrared thermography for identification of delamination and degradation of bridge components is well documented in academic research and considered a complementary step to traditional visual inspection approaches.[9]
Total station Leica MS60 measuring bridge elements
Retroreflector prism used for the measurement of distances and angles with total station
Point cloud acquisition with a laser scanner FARO.
Cost and construction management
An accurate implementation of a virtual digital twin or a BIM model of a structure is considered the starting point for budget management and optimization since the early stage studies on BMS. For example, it provides the opportunity to calculate the total cost materials and specialized operator needed in the construction step, quantifying in advance the expenses and consequently adopting better economic strategies.[10] Moreover, a multi-temporal management of information referenced to specific portions of the bridge enables the possibility to define efficient time tables for material delivery planning, project progress monitoring and documentation, construction schedule improvement and workers and experts coordination.[11] In recent BMS applications, sustainability also plays a crucial role in the definition of procedures for cost optimization adopting dedicated approaches such as Life Cycle Assessment, calculation of carbon-footprint and energy consumption along the different phase of the bridge life cycle.[8]
Structural analysis and assessment
Visual inspection often result in large amounts of data stored in the BMS inventory that serve as input for image-based processes for defect and damage detection. While traditional method for simply relied on human evaluation, Computer Vision techniques taking advantage of Artificial Intelligence and Machine Learning semi-automatize the extraction of meaningful information from pictures taken during inspections.[12] For example, recent applications of semantic segmentation allows the identification of elements affected by corrosion or other degradation phenomena,[13] enabling experts to assign a grade of severity for the damage.[1] Additional insights on the structure conditions are given by numerical simulations on fatigue behavior with finite element method modeling. This case is particularly valuable when data from in-depth detailed inspections or load tests are available, providing a rich information inventory also for computing simulations on stress behaviors and mechanics.[14][1]
At a larger territorial scale, a similar grading approach is also applied to the evaluation of the whole road network context in which the single structure analyzed is located. Such quantitative analysis are usually connected to the evaluation of road surface deformations with InSAR technologies or to calculation and prediction of the average daily traffic flow in GIS environments.[15][16]
All the results coming from analysis, simulations and severity level classifications serve as input for the execution of the intervention prioritization, the core part of the maintenance planning component in a BMS framework.[4][1]
Maintenance planning
The definition of operation schedule and more detailed inspection is a key function in the decision making process of a BMS. Based on quantitative and qualitative data acquired during routine inspections and along the processing of information in the structural analyze phase, BMS users need to identify priority interventions through a dedicated maintenance plan. This goal is achieved with the implementation of platforms and tools that enable stakeholders to explore data, results and observations and link them to detailed fact sheets reporting the health conditions of each structural elements of the bridge.[4]
Prioritization of intervention on single elements or on the whole structure is determined through a multi-criteria approach that consider the risk of defect or collapse. In particular, the process usually implies the computation of indexes for quantifying hazard, vulnerability and value exposure and derives from them a warning class. [7] Warning classes then serve as parameters for prioritizing the allocation of funds and operators for further detailed and more frequent monitoring approaches for structures at risk. As a result, bridges whose structural integrity and serviceability are more affected are classified in higher warning classes, requiring targeted interventions. This operation is essential to determine if special inspections with expert operators and specific tests (e.g. load tests) are required and if any new or additional sensors (e.g. extensometer, accelerometer) for continuous monitoring need to be installed on the structures for targeted monitoring.[7]
National guidelines
In order to assess and quantify the health condition of the bridges located in their national territory, many countries have formulated a series of general indications and guidelines for the implementation of dedicated bridge management systems.
France
In 2019, the French Centre for Studies on Risks, the Environment, Mobility and Urban Planning (CEREMA) in collaboration with the French Institute of Science and Technology for Transport, Development and Networks issued the national guidelines, proposing a multilevel methodology for the assessment and management of the risk of failure due to scour for bridges with foundations in water. The current version of the French guidelines only refers to bridge scour and hydraulic risk.The proposed methodology runs on 4 levels:[5]
- Summary analysis: qualitative risk analysis on a large scale and classification of the structure into three risk classes: low, medium, and high;
- Simplified analysis: semi-quantitative analysis on bridges previously classified in the medium and high level of risk;
- Detailed analysis: in-depth studies on high-risk structures with numerical modeling approaches
- Risk management: identification of actions to improve the conditions and/or reduce the sensitivity of critical bridges.
Italy
Ensuring the safety and well-being of these road infrastructures has become an urgent matter in Italy, especially after the evidence of bridge collapses occurred in the last decade.[17] In response to the need for reliable and up-to-date information regarding bridge conditions, in 2020 the Italian Superior Council of Public Works has developed the Guidelines on Risk Classification and Management.[18] These guidelines establish a multi-level approach for documenting bridge characteristics, assessing their health through visual inspection and damage identification, and determining their risk classification based on hazard, exposure and vulnerability derived from the previous steps.[5] Subsequently, depending on the assigned class, the number of investigation levels required to evaluate the structure's safety is determined. Road asset managers are then asked to establish and maintain a management system able to track in time interventions, documenting defects assessed on different portion of the bridge as well as environmental site conditions (hydraulics, geology, seismology). The guidelines identifies six levels:[7]
- 1. Collection of available data about bridge construction, accessing existing archives;
- 2. Visual inspection reports about structure geometry and bridge elements conditions;
- 3. Risk classification of the structure in one of the five attention classes, i.e., low, medium-low, medium, medium-high, and high;
- 4. Simplified safety assessment for bridges in medium or medium-high attention class;
- 5. Accurate safety assessment for bridges in the high attention class;
- 6. Resilience analysis at the network level. (Only drafted in the current version of the guidelines).[5]
Examples
The below are examples of commonly used bridge management software:
- Pontis, now Known as AASHTOWare Bridge Management, a BMS software sponsored by the U.S. Federal Highway Administration for the management of highway networks;[19]
- DANBRO+, computer-based BMS commonly used in Denmark;[20]
- SwissInspect, Swiss digital twin platform specialized in the management of civil infrastructures, mainly bridges;[21]
- INBEE, digital platform and mobile application that implement the Italian guidelines for bridge monitoring.[22]
See also
- Structural health monitoring
- Management system
- Digital twin
- Structural engineering
- Glossary of structural engineering
References
- ↑ 1.0 1.1 1.2 1.3 1.4 1.5 1.6 1.7 Dayan, Vandad; Chileshe, Nicholas; Hassanli, Reza (2022). "A Scoping Review of Information-Modeling Development in Bridge Management Systems" (in en). Journal of Construction Engineering and Management 148 (9). doi:10.1061/(ASCE)CO.1943-7862.0002340. ISSN 0733-9364. https://ascelibrary.org/doi/10.1061/%28ASCE%29CO.1943-7862.0002340.
- ↑ 2.0 2.1 Hawk, Hugh; Small, Edgar P. (1998). "The BRIDGIT Bridge Management System" (in en). Structural Engineering International 8 (4): 309–314. doi:10.2749/101686698780488712. ISSN 1016-8664. https://www.tandfonline.com/doi/full/10.2749/101686698780488712.
- ↑ Hudson, S. W.; Carmichael III, R. F.; Moser, L. O.; Hudson, W. R.; Wilkes, W. J. (1987). "BRIDGE MANAGEMENT SYSTEMS". NCHRP Report (300). ISSN 0077-5614. https://trid.trb.org/view/278332.
- ↑ 4.0 4.1 4.2 4.3 Mohammadi, Masoud; Rashidi, Maria; Mousavi, Vahid; Yu, Yang; Samali, Bijan (2022). "Application of TLS Method in Digitization of Bridge Infrastructures: A Path to BrIM Development" (in en). Remote Sensing 14 (5): 1148. doi:10.3390/rs14051148. ISSN 2072-4292.
- ↑ 5.0 5.1 5.2 5.3 5.4 Giordano, P.F.; Turksezer, Z.I.; Limongelli, M.P. (2022-06-27), "Risk-based scour assessment of bridges: Italian VS French guidelines" (in en), Bridge Safety, Maintenance, Management, Life-Cycle, Resilience and Sustainability (London: CRC Press): pp. 1393–1399, doi:10.1201/9781003322641-169, ISBN 978-1-003-32264-1, https://www.taylorfrancis.com/books/9781003322641/chapters/10.1201/9781003322641-169, retrieved 2023-07-05
- ↑ 6.0 6.1 Thompson, Paul D.; Small, Edgar P.; Johnson, Michael; Marshall, Allen R. (1998). "The Pontis Bridge Management System" (in en). Structural Engineering International 8 (4): 303–308. doi:10.2749/101686698780488758. ISSN 1016-8664. https://www.tandfonline.com/doi/full/10.2749/101686698780488758.
- ↑ 7.0 7.1 7.2 7.3 Natali, Agnese; Cosentino, Antonella; Morelli, Francesco; Salvatore, Walter (2023). "Multilevel Approach for Management of Existing Bridges: Critical Analysis and Application of the Italian Guidelines with the New Operating Instructions" (in en). Infrastructures 8 (4): 70. doi:10.3390/infrastructures8040070. ISSN 2412-3811.
- ↑ 8.0 8.1 Kaewunruen, Sakdirat; Sresakoolchai, Jessada; Zhou, Zhihao (2020). "Sustainability-Based Lifecycle Management for Bridge Infrastructure Using 6D BIM" (in en). Sustainability 12 (6): 2436. doi:10.3390/su12062436. ISSN 2071-1050.
- ↑ Abdallah, Abdelrahman M.; Atadero, Rebecca A.; Ozbek, Mehmet E. (2022). "A State-of-the-Art Review of Bridge Inspection Planning: Current Situation and Future Needs" (in en). Journal of Bridge Engineering 27 (2). doi:10.1061/(ASCE)BE.1943-5592.0001812. ISSN 1084-0702. https://ascelibrary.org/doi/10.1061/%28ASCE%29BE.1943-5592.0001812.
- ↑ Orcesi, André D.; Frangopol, Dan M. (2010). "Optimization of Bridge Management under Budget Constraints: Role of Structural Health Monitoring" (in en). Transportation Research Record: Journal of the Transportation Research Board 2202 (1): 148–158. doi:10.3141/2202-18. ISSN 0361-1981. http://journals.sagepub.com/doi/10.3141/2202-18.
- ↑ Zou, Yang; Kiviniemi, Arto; Jones, Stephen W.; Walsh, James (2019-02-01). "Risk Information Management for Bridges by Integrating Risk Breakdown Structure into 3D/4D BIM" (in en). KSCE Journal of Civil Engineering 23 (2): 467–480. doi:10.1007/s12205-018-1924-3. ISSN 1976-3808. https://doi.org/10.1007/s12205-018-1924-3.
- ↑ Chen, Jieh-Haur; Su, Mu-Chun; Cao, Ruijun; Hsu, Shu-Chien; Lu, Jin-Chun (2017-01-01). "A self organizing map optimization based image recognition and processing model for bridge crack inspection" (in en). Automation in Construction 73: 58–66. doi:10.1016/j.autcon.2016.08.033. ISSN 0926-5805. https://www.sciencedirect.com/science/article/pii/S092658051630187X.
- ↑ Ahuja, Sanjay Kumar; Shukla, Manoj Kumar (2018). "A Survey of Computer Vision Based Corrosion Detection Approaches". in Satapathy, Suresh Chandra; Joshi, Amit (in en). Information and Communication Technology for Intelligent Systems (ICTIS 2017) - Volume 2. Smart Innovation, Systems and Technologies. 84. Cham: Springer International Publishing. pp. 55–63. doi:10.1007/978-3-319-63645-0_6. ISBN 978-3-319-63645-0. https://link.springer.com/chapter/10.1007/978-3-319-63645-0_6.
- ↑ Zhu, Zhiwen; Xiang, Ze; Li, Jianpeng; Huang, Yan; Ruan, Shipeng (2020-04-15). "Fatigue behavior of orthotropic bridge decks with two types of cutout geometry based on field monitoring and FEM analysis" (in en). Engineering Structures 209: 109926. doi:10.1016/j.engstruct.2019.109926. ISSN 0141-0296. https://www.sciencedirect.com/science/article/pii/S0141029619310363.
- ↑ Macchiarulo, Valentina; Milillo, Pietro; Blenkinsopp, Chris; Giardina, Giorgia (2022). "Monitoring deformations of infrastructure networks: A fully automated GIS integration and analysis of InSAR time-series" (in en). Structural Health Monitoring 21 (4): 1849–1878. doi:10.1177/14759217211045912. ISSN 1475-9217. http://journals.sagepub.com/doi/10.1177/14759217211045912.
- ↑ Jiang, B.; Liu, C. (2009). "Street‐based topological representations and analyses for predicting traffic flow in GIS" (in en). International Journal of Geographical Information Science 23 (9): 1119–1137. doi:10.1080/13658810701690448. ISSN 1365-8816. https://www.tandfonline.com/doi/full/10.1080/13658810701690448.
- ↑ Calvi, Gian Michele; Moratti, Matteo; O'Reilly, Gerard J.; Scattarreggia, Nicola; Monteiro, Ricardo; Malomo, Daniele; Calvi, Paolo Martino; Pinho, Rui (2019-04-03). "Once upon a Time in Italy: The Tale of the Morandi Bridge" (in en). Structural Engineering International 29 (2): 198–217. doi:10.1080/10168664.2018.1558033. ISSN 1016-8664. https://www.tandfonline.com/doi/full/10.1080/10168664.2018.1558033.
- ↑ Consiglio Superiore dei Lavori Pubblici (2020). "Linee Guida per la classificazione e gestione del rischio, la valutazione della sicurezza ed il monitoraggio dei ponti esistenti". https://www.mit.gov.it/normativa/decreto-ministeriale-numero-204-del-1-luglio-2022.
- ↑ "AASHTOWare Bridge" (in en-US). https://www.aashtowarebridge.com/.
- ↑ Neves, Paulo J. da Sousa Cruz, Dan M. Frangopol, Luis C. Canhoto, ed (2015-03-11). Advances in Bridge Maintenance, Safety Management, and Life-Cycle Performance, Set of Book & CD-ROM: Proceedings of the Third International Conference on Bridge Maintenance, Safety and Management, 16-19 July 2006, Porto, Portugal - IABMAS '06. London: CRC Press. doi:10.1201/b18175. ISBN 978-0-429-15809-4. https://www.taylorfrancis.com/books/edit/10.1201/b18175/advances-bridge-maintenance-safety-management-life-cycle-performance-set-book-cd-rom-luis-canhoto-neves-paulo-da-sousa-cruz-dan-frangopol.
- ↑ "SwissInspect - Create Digital Twins of Infrastructure". https://www.swissinspect.io/.
- ↑ "INBEE" (in it-IT). https://inbee.it/.
Further reading
- Hudson, S. W.; Carmichael III, R. F.; Moser, L. O.; Hudson, W. R.; Wilkes, W. J. (December 1987). "BRIDGE MANAGEMENT SYSTEMS" (in en). NCHRP Report (300). https://trid.trb.org/view/278332. Retrieved 2023-07-07.
- "Linee Guida per la classificazione e gestione del rischio, la valutazione della sicurezza ed il monitoraggio dei ponti esistenti" (in it). https://www.mit.gov.it/comunicazione/news/mit-approvate-le-linee-guida-per-la-sicurezza-dei-ponti.
- "Analyse de risque des ponts en site affouillable: un guide du Cerema" (in fr). 2019-03-28. https://www.cerema.fr/fr/actualites/analyse-risque-ponts-site-affouillable-guide-du-cerema.
![]() | Original source: https://en.wikipedia.org/wiki/Bridge management system.
Read more |