Generative science
Generative science is an area of research that explores the natural world and its complex behaviours. It explores ways "to generate apparently unanticipated and infinite behaviour based on deterministic and finite rules and parameters reproducing or resembling the behavior of natural and social phenomena".[1] By modelling such interactions, it can suggest that properties exist in the system that had not been noticed in the real world situation.[2] An example field of study is how unintended consequences arise in social processes.
Generative sciences often explore natural phenomena at several levels of organization.[3][4] Self-organizing natural systems are a central subject, studied both theoretically and by simulation experiments. The study of complex systems in general has been grouped under the heading of "general systems theory", particularly by Ludwig von Bertalanffy, Anatol Rapoport, Ralph Gerard, and Kenneth Boulding.
Scientific and philosophical origins
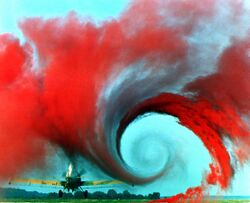

The development of computers and automata theory laid a technical foundation for the growth of the generative sciences. For example:
- Cellular automata are mathematical representations of simple entities interacting under deterministic rules to manifest complex behaviours. They can be used to model emergent processes of the physical universe, neural cognitive processes and social behavior.[6][7][8][9]
- Conway's Game of Life is a zero-player game based on cellular automata, meaning that the only input is in setting the initial conditions, and the game is to see how the system evolves.[10]
- In 1996 Joshua M. Epstein and Robert Axtell wrote the book Growing Artificial Societies which proposes a set of automaton rules and a system called Sugarscape which models a population dependent on resources (called sugar).
- Artificial neural networks attempt to solve problems in the same way that the human brain would, although they are still several orders of magnitude less complex than the human brain and closer to the computing power of a worm. Advances in the understanding of the human brain often stimulate new patterns in neural networks.
One of the most influential advances in the generative sciences as related to cognitive science came from Noam Chomsky's (1957) development of generative grammar, which separated language generation from semantic content, and thereby revealed important questions about human language. It was also in the early 1950s that psychologists at the MIT including Kurt Lewin, Jacob Levy Moreno and Fritz Heider laid the foundations for group dynamics research which later developed into social network analysis.
See also
- Generative systems – Technologies that can produce change driven by audiences
References
- ↑ Gordana Dodig-Crnkovic; Raffaela Giovagnoli (2013), "Computing Nature – A Network of Networks of Concurrent Information Processes", in Gordana Dodig-Crnkovic; Raffaela Giovagnoli, Computing nature: Turing centenary perspective, Springer, p. 7, ISBN 978-3-642-37225-4
- ↑ Ning Nan; Erik W. Johnston; Judith S. Olson (2008), "Unintended consequences of collocation: using agent-based modeling to untangle effects of communication delay and in-group favor", Computational and Mathematical Organization Theory 14 (2): 57–83, doi:10.1007/s10588-008-9024-4
- ↑ Farre, G. L. (1997). "The Energetic Structure of Observation: A Philosophical Disquisition". American Behavioral Scientist 40 (6): 717–728. doi:10.1177/0002764297040006004.
- ↑ J. Schmidhuber. (1997) A computer scientist's view of life, the universe, and everything. Foundations of Computer Science: Potential – Theory – Cognition, Lecture Notes in Computer Science, pages 201–208, Springer
- ↑ Hermann Cuntz (2010). "PLoS Computational Biology Issue Image | Vol. 6(8) August 2010". PLOS Computational Biology 6 (8): ev06.ei08. doi:10.1371/image.pcbi.v06.i08.
- ↑ Kenrick, DT; Li, NP; Butner, J (2003). "Dynamical evolutionary psychology: individual decision rules and emergent social norms". Psychological Review 110 (1): 3–28. doi:10.1037/0033-295X.110.1.3. PMID 12529056.
- ↑ Epstein, Joshua M.; Axtell, Robert L. (1996). Growing Artificial Societies: Social Science From the Bottom Up. Cambridge MA: MIT/Brookings Institution. p. 224. ISBN 978-0-262-55025-3. https://archive.org/details/growingartificia00epst/page/224.
- ↑ Nowak A.; Vallacher R.R.; Tesser A.; Borkowski W. (2000), "Society of Self: The emergence of collective properties in self-structure", Psychological Review 107 (1): 39–61, doi:10.1037/0033-295x.107.1.39, PMID 10687402
- ↑ Epstein J.M. (1999), "Agent Based Computational Models and Generative Social Science", Complexity 4 (5): 41–60, doi:10.1002/(SICI)1099-0526(199905/06)4:5<41::AID-CPLX9>3.0.CO;2-F, Bibcode: 1999Cmplx...4e..41E
- ↑ John Conway's Game of Life
External links
- http://www.swarthmore.edu/socsci/tburke1/artsoc.html (Artificial Societies, Virtual Worlds and the Shared Problems and Possibilities of Emergence)
- http://jasss.soc.surrey.ac.uk/JASSS.html (The Journal of Artificial Societies and Social Simulation)
![]() | Original source: https://en.wikipedia.org/wiki/Generative science.
Read more |