Moment problem
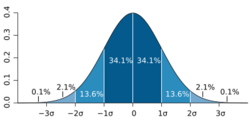
In mathematics, a moment problem arises as the result of trying to invert the mapping that takes a measure [math]\displaystyle{ \mu }[/math] to the sequence of moments
- [math]\displaystyle{ m_n = \int_{-\infty}^\infty x^n \,d\mu(x)\,. }[/math]
More generally, one may consider
- [math]\displaystyle{ m_n = \int_{-\infty}^\infty M_n(x) \,d\mu(x)\,. }[/math]
for an arbitrary sequence of functions [math]\displaystyle{ M_n }[/math].
Introduction
In the classical setting, [math]\displaystyle{ \mu }[/math] is a measure on the real line, and [math]\displaystyle{ M }[/math] is the sequence [math]\displaystyle{ \{x^n : n=1,2,\dotsc\} }[/math]. In this form the question appears in probability theory, asking whether there is a probability measure having specified mean, variance and so on, and whether it is unique.
There are three named classical moment problems: the Hamburger moment problem in which the support of [math]\displaystyle{ \mu }[/math] is allowed to be the whole real line; the Stieltjes moment problem, for [math]\displaystyle{ [0,\infty) }[/math]; and the Hausdorff moment problem for a bounded interval, which without loss of generality may be taken as [math]\displaystyle{ [0,1] }[/math].
The moment problem also extends to complex analysis as the trigonometric moment problem in which the Hankel matrices are replaced by Toeplitz matrices and the support of μ is the complex unit circle instead of the real line.[1]
Existence
A sequence of numbers [math]\displaystyle{ m_n }[/math] is the sequence of moments of a measure [math]\displaystyle{ \mu }[/math] if and only if a certain positivity condition is fulfilled; namely, the Hankel matrices [math]\displaystyle{ H_n }[/math],
- [math]\displaystyle{ (H_n)_{ij} = m_{i+j}\,, }[/math]
should be positive semi-definite. This is because a positive-semidefinite Hankel matrix corresponds to a linear functional [math]\displaystyle{ \Lambda }[/math] such that [math]\displaystyle{ \Lambda(x^n) = m_n }[/math] and [math]\displaystyle{ \Lambda(f^2) \geq 0 }[/math] (non-negative for sum of squares of polynomials). Assume [math]\displaystyle{ \Lambda }[/math] can be extended to [math]\displaystyle{ \mathbb{R}[x]^* }[/math]. In the univariate case, a non-negative polynomial can always be written as a sum of squares. So the linear functional [math]\displaystyle{ \Lambda }[/math] is positive for all the non-negative polynomials in the univariate case. By Haviland's theorem, the linear functional has a measure form, that is [math]\displaystyle{ \Lambda(x^n) = \int_{-\infty}^{\infty} x^n d \mu }[/math]. A condition of similar form is necessary and sufficient for the existence of a measure [math]\displaystyle{ \mu }[/math] supported on a given interval [math]\displaystyle{ [a,b] }[/math].
One way to prove these results is to consider the linear functional [math]\displaystyle{ \varphi }[/math] that sends a polynomial
- [math]\displaystyle{ P(x) = \sum_k a_k x^k }[/math]
to
- [math]\displaystyle{ \sum_k a_k m_k. }[/math]
If [math]\displaystyle{ m_k }[/math] are the moments of some measure [math]\displaystyle{ \mu }[/math] supported on [math]\displaystyle{ [a,b] }[/math], then evidently
-
[math]\displaystyle{ \varphi(P) \ge 0 }[/math] for any polynomial [math]\displaystyle{ P }[/math] that is non-negative on [math]\displaystyle{ [a,b] }[/math].
(
)
-
Vice versa, if (1) holds, one can apply the M. Riesz extension theorem and extend [math]\displaystyle{ \varphi }[/math] to a functional on the space of continuous functions with compact support [math]\displaystyle{ C_c([a,b]) }[/math]), so that
-
[math]\displaystyle{ \varphi(f) \ge 0 }[/math] for any [math]\displaystyle{ f \in C_c([a,b]),\;f\ge 0. }[/math]
(
)
-
By the Riesz representation theorem, (2) holds iff there exists a measure [math]\displaystyle{ \mu }[/math] supported on [math]\displaystyle{ [a,b] }[/math], such that
- [math]\displaystyle{ \varphi(f) = \int f \, d\mu }[/math]
for every [math]\displaystyle{ f \in C_c([a,b]) }[/math].
Thus the existence of the measure [math]\displaystyle{ \mu }[/math] is equivalent to (1). Using a representation theorem for positive polynomials on [math]\displaystyle{ [a,b] }[/math], one can reformulate (1) as a condition on Hankel matrices.[2][3]
Uniqueness (or determinacy)
The uniqueness of [math]\displaystyle{ \mu }[/math] in the Hausdorff moment problem follows from the Weierstrass approximation theorem, which states that polynomials are dense under the uniform norm in the space of continuous functions on [math]\displaystyle{ [0,1] }[/math]. For the problem on an infinite interval, uniqueness is a more delicate question.[4] There are distributions, such as log-normal distributions, which have finite moments for all the positive integers but where other distributions have the same moments.
Formal solution
When the solution exists, it can be formally written using derivatives of the Dirac delta function as
- [math]\displaystyle{ d\mu(x) = \rho(x) dx, \quad \rho(x) = \sum_{n=0}^\infty \frac{(-1)^n}{n!}\delta^{(n)}(x)m_n }[/math].
The expression can be derived by taking the inverse Fourier transform of its characteristic function.
Variations
An important variation is the truncated moment problem, which studies the properties of measures with fixed first k moments (for a finite k). Results on the truncated moment problem have numerous applications to extremal problems, optimisation and limit theorems in probability theory.[3]
Probability
The moment problem has applications to probability theory. The following is commonly used:[5]
Theorem (Fréchet-Shohat) — If [math]\displaystyle{ \mu }[/math] is a determinate measure (i.e. its moments determine it uniquely), and the measures [math]\displaystyle{ \mu_n }[/math] are such that [math]\displaystyle{ \forall k \geq 0 \quad \lim _{n \rightarrow \infty} m_k\left[\mu_n\right]=m_k[\mu], }[/math] then [math]\displaystyle{ \mu_n \rightarrow \mu }[/math] in distribution.
By checking Carleman's condition, we know that the standard normal distribution is a determinate measure, thus we have the following form of the central limit theorem:
Corollary — If a sequence of probability distributions [math]\displaystyle{ \nu_n }[/math] satisfy [math]\displaystyle{ m_{2k}[\nu_n] \to \frac{(2k)!}{2^k k!}; \quad m_{2k+1}[\nu_n] \to 0 }[/math] then [math]\displaystyle{ \nu_n }[/math] converges to [math]\displaystyle{ N(0, 1) }[/math] in distribution.
See also
- Carleman's condition
- Hamburger moment problem
- Hankel matrix
- Hausdorff moment problem
- Moment (mathematics)
- Stieltjes moment problem
- Trigonometric moment problem
Notes
- ↑ Schmüdgen 2017, p. 257.
- ↑ Shohat & Tamarkin 1943.
- ↑ 3.0 3.1 Kreĭn & Nudel′man 1977.
- ↑ Akhiezer 1965.
- ↑ Sodin, Sasha (March 5, 2019). "The classical moment problem". https://webspace.maths.qmul.ac.uk/a.sodin/teaching/moment/clmp.pdf.
References
- Shohat, James Alexander; Tamarkin, Jacob D. (1943). The Problem of Moments. New York: American mathematical society. ISBN 978-1-4704-1228-9.
- Akhiezer, Naum I. (1965). The classical moment problem and some related questions in analysis. New York: Hafner Publishing Co.. https://archive.org/details/classicalmomentp0000akhi. (translated from the Russian by N. Kemmer)
- Kreĭn, M. G.; Nudel′man, A. A. (1977). The Markov Moment Problem and Extremal Problems. Translations of Mathematical Monographs. Providence, Rhode Island: American Mathematical Society. doi:10.1090/mmono/050. ISBN 978-0-8218-4500-4.
- Schmüdgen, Konrad (2017). The Moment Problem. Graduate Texts in Mathematics. Cham: Springer International Publishing. doi:10.1007/978-3-319-64546-9. ISBN 978-3-319-64545-2.
![]() | Original source: https://en.wikipedia.org/wiki/Moment problem.
Read more |