Transport network
Network science | ||||
---|---|---|---|---|
Network types | ||||
Graphs | ||||
|
||||
Models | ||||
|
||||
| ||||
A transport network, or transportation network is a realisation of a spatial network, describing a structure which permits either vehicular movement or flow of some commodity.[1] Examples include but are not limited to road networks, railways, air routes, pipelines, aqueducts, and power lines.
Methods
Transport network analysis is used to determine the flow of vehicles (or people) through a transport network, typically using mathematical graph theory. It may combine different modes of transport, for example, walking and car, to model multi-modal journeys. Transport network analysis falls within the field of transport engineering. Traffic has been studied extensively using statistical physics methods.[2][3][4] Recently a real transport network of Beijing was studied using a network approach and percolation theory. The research showed that one can characterize the quality of global traffic in a city at each time in the day using percolation threshold, see Fig. 1. In recent articles, percolation theory has been applied to study traffic congestion in a city. The quality of the global traffic in a city at a given time is by a single parameter, the percolation critical threshold. The critical threshold represents the velocity below which one can travel in a large fraction of city network. The method is able to identify repetitive traffic bottlenecks. [5] Critical exponents characterizing the cluster size distribution of good traffic are similar to those of percolation theory.[6]
An empirical study regarding the size distribution of traffic jams has been performed recently by Zhang et al. [7] They found an approximate universal power law for the jam sizes distribution.
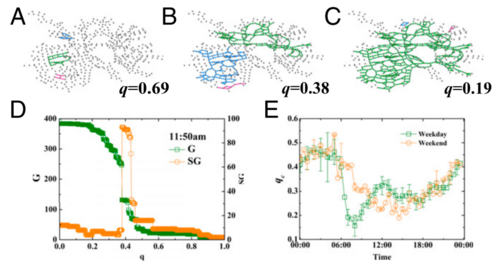
See also
- Braess' paradox
- Flow network
- Heuristic routing
- Interplanetary Transport Network
- Network science
- Percolation theory
- Street network
- Rail network
- Multimodal transport
References
- ↑ Barthelemy, Marc (2010). "Spatial Networks". Physics Reports 499 (1–3): 1–101. doi:10.1016/j.physrep.2010.11.002. Bibcode: 2011PhR...499....1B.
- ↑ Helbing, D (2001). "Traffic and related self-driven many-particle systems". Reviews of Modern Physics 73 (4): 1067–1141. doi:10.1103/RevModPhys.73.1067. Bibcode: 2001RvMP...73.1067H.
- ↑ S., Kerner, Boris (2004). The Physics of Traffic : Empirical Freeway Pattern Features, Engineering Applications, and Theory. Berlin, Heidelberg: Springer Berlin Heidelberg. ISBN 9783540409861. OCLC 840291446.
- ↑ Wolf, D E; Schreckenberg, M; Bachem, A (June 1996) (in en-US). Traffic and Granular Flow. WORLD SCIENTIFIC. 1–394. doi:10.1142/9789814531276. ISBN 9789810226350.
- ↑ Li, Daqing; Fu, Bowen; Wang, Yunpeng; Lu, Guangquan; Berezin, Yehiel; Stanley, H. Eugene; Havlin, Shlomo (2015-01-20). "Percolation transition in dynamical traffic network with evolving critical bottlenecks" (in en). Proceedings of the National Academy of Sciences 112 (3): 669–672. doi:10.1073/pnas.1419185112. ISSN 0027-8424. PMID 25552558. Bibcode: 2015PNAS..112..669L.
- ↑ Switch between critical percolation modes in city traffic dynamics G Zeng, D Li, S Guo, L Gao, Z Gao, HE Stanley, S Havlin Proceedings of the National Academy of Sciences 116 (1), 23-28 (2019) Scale-free resilience of real traffic jams
- ↑ Scale-free resilience of real traffic jams Limiao Zhang, Guanwen Zeng, Daqing Li, Hai-Jun Huang, H Eugene Stanley, Shlomo Havlin Proceedings of the National Academy of Sciences 116(18), 8673-8678 (2019)