Multivariate analysis of variance
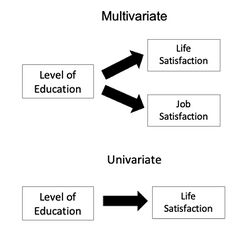
In statistics, multivariate analysis of variance (MANOVA) is a procedure for comparing multivariate sample means. As a multivariate procedure, it is used when there are two or more dependent variables,[1] and is often followed by significance tests involving individual dependent variables separately.[2]
Without relation to the image, the dependent variables may be k life satisfactions scores measured at sequential time points and p job satisfaction scores measured at sequential time points. In this case there are k+p dependent variables whose linear combination follows a multivariate normal distribution, multivariate variance-covariance matrix homogeneity, and linear relationship, no multicollinearity, and each without outliers.
Model
Assume [math]\displaystyle{ n }[/math] [math]\displaystyle{ q }[/math]-dimensional observations, where the [math]\displaystyle{ i }[/math]’th observation [math]\displaystyle{ y_i }[/math] is assigned to the group [math]\displaystyle{ g(i)\in \{1,\dots,m\} }[/math] and is distributed around the group center [math]\displaystyle{ \mu^{(g(i))}\in \mathbb R^q }[/math] with multivariate Gaussian noise: [math]\displaystyle{ y_i = \mu^{(g(i))} + \varepsilon_i\quad \varepsilon_i \overset{\text{i.i.d.}}{\sim} \mathcal N_q (0, \Sigma) \quad \text{ for } i=1,\dots, n, }[/math] where [math]\displaystyle{ \Sigma }[/math] is the covariance matrix. Then we formulate our null hypothesis as [math]\displaystyle{ H_0\!:\;\mu^{(1)}=\mu^{(2)}=\dots =\mu^{(m)}. }[/math]
Relationship with ANOVA
MANOVA is a generalized form of univariate analysis of variance (ANOVA),[1] although, unlike univariate ANOVA, it uses the covariance between outcome variables in testing the statistical significance of the mean differences.
Where sums of squares appear in univariate analysis of variance, in multivariate analysis of variance certain positive-definite matrices appear. The diagonal entries are the same kinds of sums of squares that appear in univariate ANOVA. The off-diagonal entries are corresponding sums of products. Under normality assumptions about error distributions, the counterpart of the sum of squares due to error has a Wishart distribution.
Hypothesis Testing
First, define the following [math]\displaystyle{ n\times q }[/math] matrices:
- [math]\displaystyle{ Y }[/math]: where the [math]\displaystyle{ i }[/math]-th row is equal to [math]\displaystyle{ y_i }[/math]
- [math]\displaystyle{ \hat Y }[/math]: where the [math]\displaystyle{ i }[/math]-th row is the best prediction given the group membership [math]\displaystyle{ g(i) }[/math]. That is the mean over all observation in group [math]\displaystyle{ g(i) }[/math]: [math]\displaystyle{ \frac{1}{\text{size of group }g(i)}\sum_{k: g(k)=g(i)}y_k }[/math].
- [math]\displaystyle{ \bar Y }[/math]: where the [math]\displaystyle{ i }[/math]-th row is the best prediction given no information. That is the empirical mean over all [math]\displaystyle{ n }[/math] observations [math]\displaystyle{ \frac{1}{n}\sum_{k=1}^n y_k }[/math]
Then the matrix [math]\displaystyle{ S_{\text{model}} := (\hat Y - \bar Y)^T(\hat Y - \bar Y) }[/math] is a generalization of the sum of squares explained by the group, and [math]\displaystyle{ S_{\text{res}} := (Y - \hat Y)^T(Y - \hat Y) }[/math] is a generalization of the residual sum of squares.[3] [4] Note that alternatively one could also speak about covariances when the abovementioned matrices are scaled by 1/(n-1) since the subsequent test statistics do not change by multiplying [math]\displaystyle{ S_{\text{model}} }[/math] and [math]\displaystyle{ S_{\text{res}} }[/math] by the same non-zero constant.
The most common[5][6] statistics are summaries based on the roots (or eigenvalues) [math]\displaystyle{ \lambda_p }[/math] of the matrix [math]\displaystyle{ A:= S_{\text{model}}S_{\text{res}}^{-1} }[/math]
- Samuel Stanley Wilks' [math]\displaystyle{ \Lambda_\text{Wilks} = \prod_{1,\ldots,p}(1/(1 + \lambda_{p})) = \det(I + A)^{-1} = \det(S_\text{res})/\det(S_\text{res} + S_\text{model}) }[/math] distributed as lambda (Λ)
- the K. C. Sreedharan Pillai–M. S. Bartlett trace, [math]\displaystyle{ \Lambda_\text{Pillai} = \sum_{1,\ldots,p}(\lambda_p/(1 + \lambda_p)) = \operatorname{tr}(A(I + A)^{-1}) }[/math][7]
- the Lawley–Hotelling trace, [math]\displaystyle{ \Lambda_\text{LH} = \sum_{1,\ldots,p}(\lambda_{p}) = \operatorname{tr}(A) }[/math]
- Roy's greatest root (also called Roy's largest root), [math]\displaystyle{ \Lambda_\text{Roy} = \max_p(\lambda_p) }[/math]
Discussion continues over the merits of each,[1] although the greatest root leads only to a bound on significance which is not generally of practical interest. A further complication is that, except for the Roy's greatest root, the distribution of these statistics under the null hypothesis is not straightforward and can only be approximated except in a few low-dimensional cases.[8] An algorithm for the distribution of the Roy's largest root under the null hypothesis was derived in [9] while the distribution under the alternative is studied in.[10]
The best-known approximation for Wilks' lambda was derived by C. R. Rao.
In the case of two groups, all the statistics are equivalent and the test reduces to Hotelling's T-square.
Introducing Covariates (MANCOVA)
One can also test if there is a group effect after adjusting for covariates. For this, follow the procedure above but substitute [math]\displaystyle{ \hat Y }[/math] with the predictions of the general linear model, containing the group and the covariates, and substitute [math]\displaystyle{ \bar Y }[/math] with the predictions of the general linear model containing only the covariates (and an intercept). Then [math]\displaystyle{ S_{\text{model}} }[/math] are the additional sum of squares explained by adding the grouping information and [math]\displaystyle{ S_{\text{res}} }[/math] is the residual sum of squares of the model containing the grouping and the covariates.[4]
Note that in case of unbalanced data, the order of adding the covariates matter.
Correlation of dependent variables

MANOVA's power is affected by the correlations of the dependent variables and by the effect sizes associated with those variables. For example, when there are two groups and two dependent variables, MANOVA's power is lowest when the correlation equals the ratio of the smaller to the larger standardized effect size.[11]
See also
- Permutational analysis of variance for a non-parametric alternative
- Discriminant function analysis
- Canonical correlation analysis
- Multivariate analysis of variance (Wikiversity)
- Repeated measures design
References
- ↑ 1.0 1.1 1.2 Warne, R. T. (2014). "A primer on multivariate analysis of variance (MANOVA) for behavioral scientists". Practical Assessment, Research & Evaluation 19 (17): 1–10. https://scholarworks.umass.edu/pare/vol19/iss1/17/.
- ↑ Stevens, J. P. (2002). Applied multivariate statistics for the social sciences. Mahwah, NJ: Lawrence Erblaum.
- ↑ Anderson, T. W. (1994). An Introduction to Multivariate Statistical Analysis. Wiley.
- ↑ 4.0 4.1 Krzanowski, W. J. (1988). Principles of Multivariate Analysis. A User's Perspective. Oxford University Press.
- ↑ Garson, G. David. "Multivariate GLM, MANOVA, and MANCOVA". http://faculty.chass.ncsu.edu/garson/PA765/manova.htm.
- ↑ UCLA: Academic Technology Services, Statistical Consulting Group.. "Stata Annotated Output – MANOVA". http://www.ats.ucla.edu/stat/stata/output/Stata_MANOVA.htm.
- ↑ "MANOVA Basic Concepts – Real Statistics Using Excel". http://www.real-statistics.com/multivariate-statistics/multivariate-analysis-of-variance-manova/manova-basic-concepts/.
- ↑ Camo http://www.camo.com/multivariate_analysis.html
- ↑ Chiani, M. (2016), "Distribution of the largest root of a matrix for Roy's test in multivariate analysis of variance", Journal of Multivariate Analysis 143: 467–471, doi:10.1016/j.jmva.2015.10.007
- ↑ I.M. Johnstone, B. Nadler "Roy's largest root test under rank-one alternatives" arXiv preprint arXiv:1310.6581 (2013)
- ↑ Frane, Andrew (2015). "Power and Type I Error Control for Univariate Comparisons in Multivariate Two-Group Designs". Multivariate Behavioral Research 50 (2): 233–247. doi:10.1080/00273171.2014.968836. PMID 26609880.
External links
![]() | Original source: https://en.wikipedia.org/wiki/Multivariate analysis of variance.
Read more |